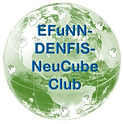
EFuNN - DENFIS - NeuCube Club
Moderator: PhD Ms Iman AbouHassan
"Unleashing the Future Applications of Advanced Artificial Intelligence and Neural Networks
in Finance and Economics" - Presentation - Aug 15, 2023
:
The Club is intended to help users of ECOS and NeuCube to share knowledge and experience for further development and applications of these important methods of contemporary AI.
Authors of papers and projects using ECOS and NeuCube are invited to contact the Moderator and to make their research available on this forum to inform the community.
-------------------------------------------------------------------------------------------------------------------------
Professor Nikola Kasabov pioneered two important theoretical and computational frameworks of hybrid explainable evolving connectionist systems (ECOS) with a significant scientific and technological impact in the area of neural networks, namely:
(1) Evolving neuro-fuzzy systems (1998-2002); (2) Brain-inspired Spiking Neural Networks (2006), along with their corresponding methods, algorithms and applications. The main methods in the first framework are EFuNN, 2001 (Evolving Fuzzy Neural Network) and DENFIS, 2002 (Dynamic Evolving Neuro-Fuzzy Inference System). The main methods in the second framework are eSNN, 2006 (Evolving spiking neural networks) and CNGM, 2006 (Computational Neuro-Genetic Modelling). The eSNN and CNGM are later used to create a popular brain-inspired SNN architecture NeuCube (2014). The methods are published and also patented, with early publications as follows:
-
Kasabov, N. Foundations of neural networks, fuzzy systems and knowledge engineering, MIT Press 1996.
-
Kasabov, N. ECOS - A framework for evolving connectionist systems and the 'eco' training method, in: S.Usui and T.Omori (eds) Proceedings of ICONIP'98 - The 5 Int. Conf. on Neural Information Processing (ICONIP), Kitakyushu, Japan, 21-23 October 1998, IOS Press, vol.3, 1232-1235
-
Kasabov N (2001) Evolving fuzzy neural networks for online supervised/unsupervised, knowledge–based learning. IEEE Trans. SMC/ B, Cybernetics 31(6): 902-918.
-
Kasabov, N., Adaptive learning system and method, Patent US 7,089,217B2, Apr.10, 2001.
-
Kasabov N, and Song Q (2002) DENFIS: Dynamic, evolving neural-fuzzy inference systems and its application for time-series prediction. IEEE Trans. on Fuzzy Systems, vol. 10:144 – 154.
-
Kasabov, N., Neuro-, genetic-, and quantum-inspired evolving intelligent systems, Proc. 2006 Int. Symposium on Evolving Fuzzy Systems, September 2006, UK, IEEE Press, 63-73, 2006.
-
Kasabov, N. Evolving Connectionist Systems: The Knowledge Engineering Approach, Springer Verlag, London, (2007) 458p.
-
Kasabov, N. Evolving Intelligence in Humans and Machines: Integrative Connectionist Systems Approach, Feature article, IEEE CIS Magazine, August 2008, vol.3, Num.3, pp. 23-37.
An ECOS was defined by Kasabov as a hybrid, neural network-based model or a system, characterized by the following principles: (1) Fast learning; (2) Adaptation in a real-time; (3) Open, evolvable structure; (4) Knowledge-based explainability; (5) Active interaction with the environment; (6) Representing both space and time; (7) Self-evaluation and optimization.
The two major evolving neuro-fuzzy methods EFuNN and DENFIS are implemented in a free NeuCom software environment (http://theneucom.com) and are used now in more than 50 labs across the world (1000+ downloads only in 2022).
The methods of eSNN and CNGM, where information is represented as spatio-temporal spike sequences rather than scalars, were used later to create a brain-inspired computational architecture for spatio-temporal data modelling, called NeuCube, implemented also as a development software (www.kedri.aut.ac.nz/neucube/) adopted in more than 35 labs across the world (1200 downloads only in 2022).
Novel applications and publications of eSNN are for brain data modelling (EEG, fMRI); seismic data modelling; brain-computer interfaces for neurorehabilitation; personalized prediction of stroke, dementia, psychosis and others, some of them covered in Kasabov’s recent book: Time-Space, Spiking Neural Networks and Brain-Inspired Artificial Intelligence, Springer Nature (2019) 750p., https://www.springer.com/gp/book/9783662577134.
The proposed ECOS methods and systems have a significant scientific and technological impact internationally. They attracted research groups and individual researchers to develop a large number of new ECOS-inspired algorithms and their engineering applications. Such ECOS-based methods, software systems and engineering application systems have been further developed by him and also by groups across countries (New Zealand, Australia, USA, the UK, Brazil, Hungary, China, Japan, Switzerland, India, Indonesia, Malaysia, Bulgaria, Turkey, Greece, Spain, Italy, Portugal, Poland, France).
Just a few examples of specific ECOS methods, software implementations and engineering applications of ECOS developed by Prof. Kasabov and his team are:
-
Discovery of diagnostic markers for early detection of bladder cancer, colorectal cancer and other types of cancer based on EFuNN (Pacific Edge Biotechnology Ltd, https://www.pacificedgedx.com);
-
Medical diagnosis of renal function evaluation using DENFIS (Marshall, M.R. et al);
-
Risk analysis and discovery of evolving economic clusters in Europe (N Kasabov, L Erzegovesi, M Fedrizzi, A Beber, 2000);
-
Adaptive robot control system based on ECOS – first prize at the Robocup in 2004, with a team from Singapore (Huang, L. et al).
-
Environmental modelling (the spread of invasive species; earthquake prediction) (2008).
-
eSNN and NeuCube-based application systems for predictive modelling of stroke (2014, 2022), dementia (2022), and psychosis (2023).
Labs across the globe implemented ECOS methods and used them for a large scope of applications, an overview of which can be found in a recent paper by Kasabov:
Examples of engineering applications explained in the above paper are:
-
Stock market prediction (Proc. IEEE IS, 2022);
-
Modelling oxygen concentration in a river;
-
Modelling coagulant dosage;
-
Rainfall water modelling: https://www.mdpi.com/2073-4441/11/1/52/htm;
-
Mortality prediction: https://www.ijera.com/papers/Vol7_issue3/Part-6/E0703062629.pdf,
-
Flood attack detection: http://www.indjst.org/index.php/indjst/article/view/47680,
-
EFuNN Ensembles: https://link.springer.com/chapter/10.1007/11893295_97.
-
Emotion classification using EFuNN: http://irep.iium.edu.my/43492.
-
Predicting response to treatment of drug addicts.
-
Early prediction of stroke.
-
Predicting the onset of dementia.